The rapid advancement of AI image generation has unlocked new creative possibilities, but many models still lack the fine-grained control that practitioners desire. In this article we introduce ControlNet, a new architecture that allow you to steer pretrained Diffusion Models.
Building on Stable Diffusion [1] architecture, ControlNet [2] demonstrates the next evolution in AI creativity, moving beyond static imagery toward controllable and customizable image generation.
In this article we introduce you this pioneering work, which recently won the Marr Prize (i.e. best paper award) at the prestigious ICCV23 conference.
Table of Contents
- ControlNet’s key idea: control is everything
- Stable Diffusion limitations
- How ControlNet generates images: the 1 min explanation
- Can we use ControlNet to augment a dataset?
- What’s next
1. ControlNet’s key idea: control is everything
ControlNet is an end-to-end architecture that learns conditional controls for large pre-trained text-to-image diffusion models [2]. Figure 1 shows the poster session of ControlNet, developed by researchers from Stanford University: Lvmin Zhang, Anyi Rao, and Maneesh Agrawala.
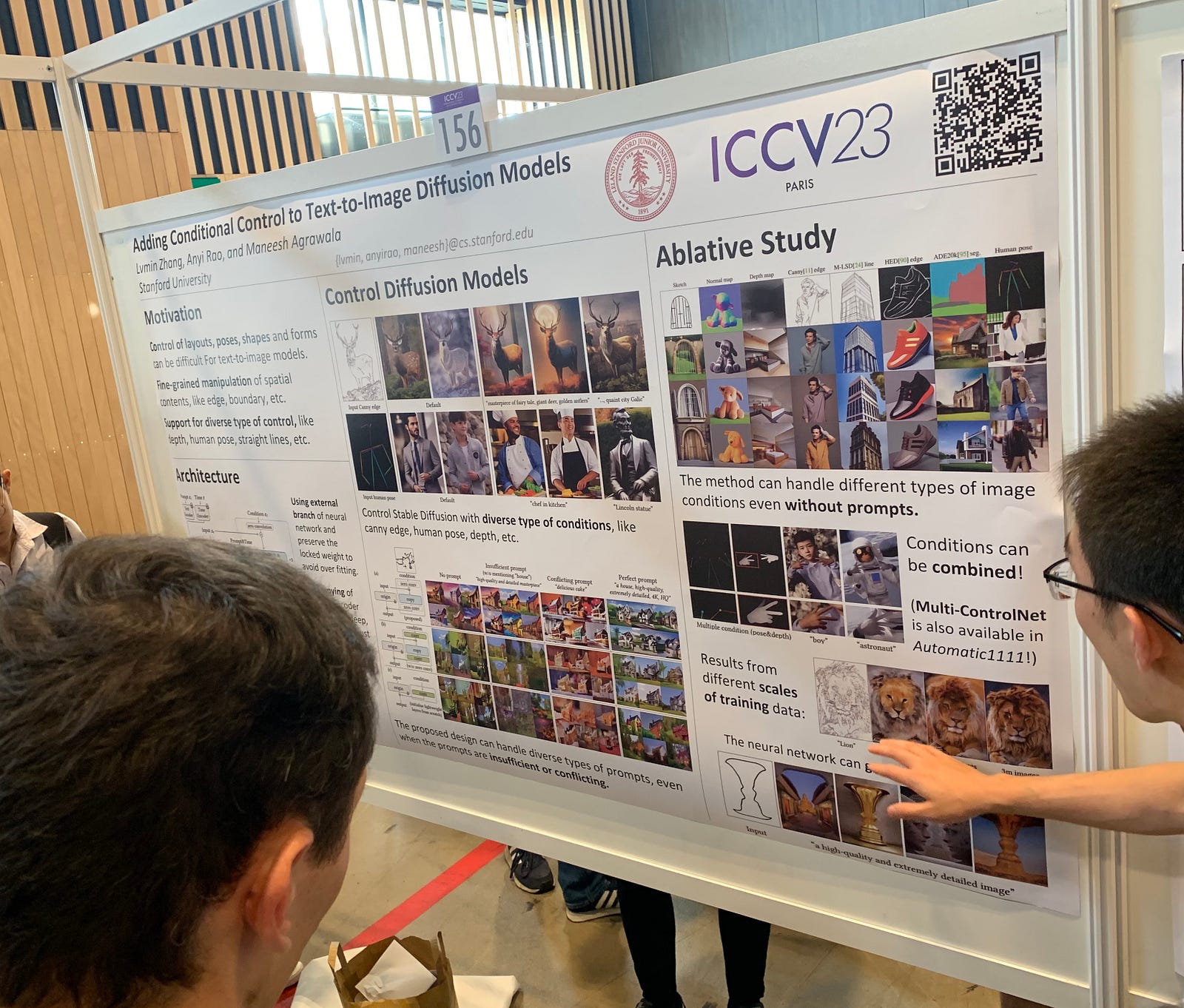
An in-depth explanation of this approach can be found on ControlNet’s paper, alternatively we summarize what makes ControlNet special in the following five points.
- 1. ControlNet leverages frozen encoding layers of diffusion models [4] to reuse robust image features.
- 2. It adds new trainable components for granular spatial conditioning controls.
- 3. ControlNet uses zero convolutions to safely add new parameters atop base model.
- 4. It teaches spatial control like pose and lighting without harming image quality.
- 5. Overall, it unlocks intentional creativity from diffusion models without impacting training.
2. Stable Diffusion limitations
While Stable Diffusion has demonstrated impressive ability to generate novel, high-quality images, it lacks granular control over important attributes in the output [2].
When using Stable Diffusion or similar models to create an image prompt, the pose, position, and structural details of objects can vary wildly between iterations [3].
Techniques like Img2Img allow guiding the style and composition to a degree, but do not enable precise direction of lighting, perspective, proportions, and other fine-grained elements.
How Img2Img approaches allow for fine-grained control
- An initial image is generated using a model like DALL-E or Stable Diffusion based on a text prompt.
- The initial image is then fed back into the model along with adjustments to the prompt. For example, “Make the person in this image smile more.”
- The model then generates a new image that retains aspects of the original image while incorporating the adjustments from the updated prompt.
- This new image can be fed back into the model again for further refinements, creating an iterative loop to reach the desired result.
Randomness is good until it is not
For many creative applications, the previous approach contains too much randomness, which reduces the utility of AI image generation for downstream tasks (i.e. reliably generating hundreds of high quality marketing images, or generating synthetic data for an imbalanced dataset).
The inability to deliberatelly modify pose, scale, angles, and structural aspects inhibits using Stable Diffusion (or any similar model) for tasks requiring predictable results.
3. How ControlNet generates images: the 1 min explanation
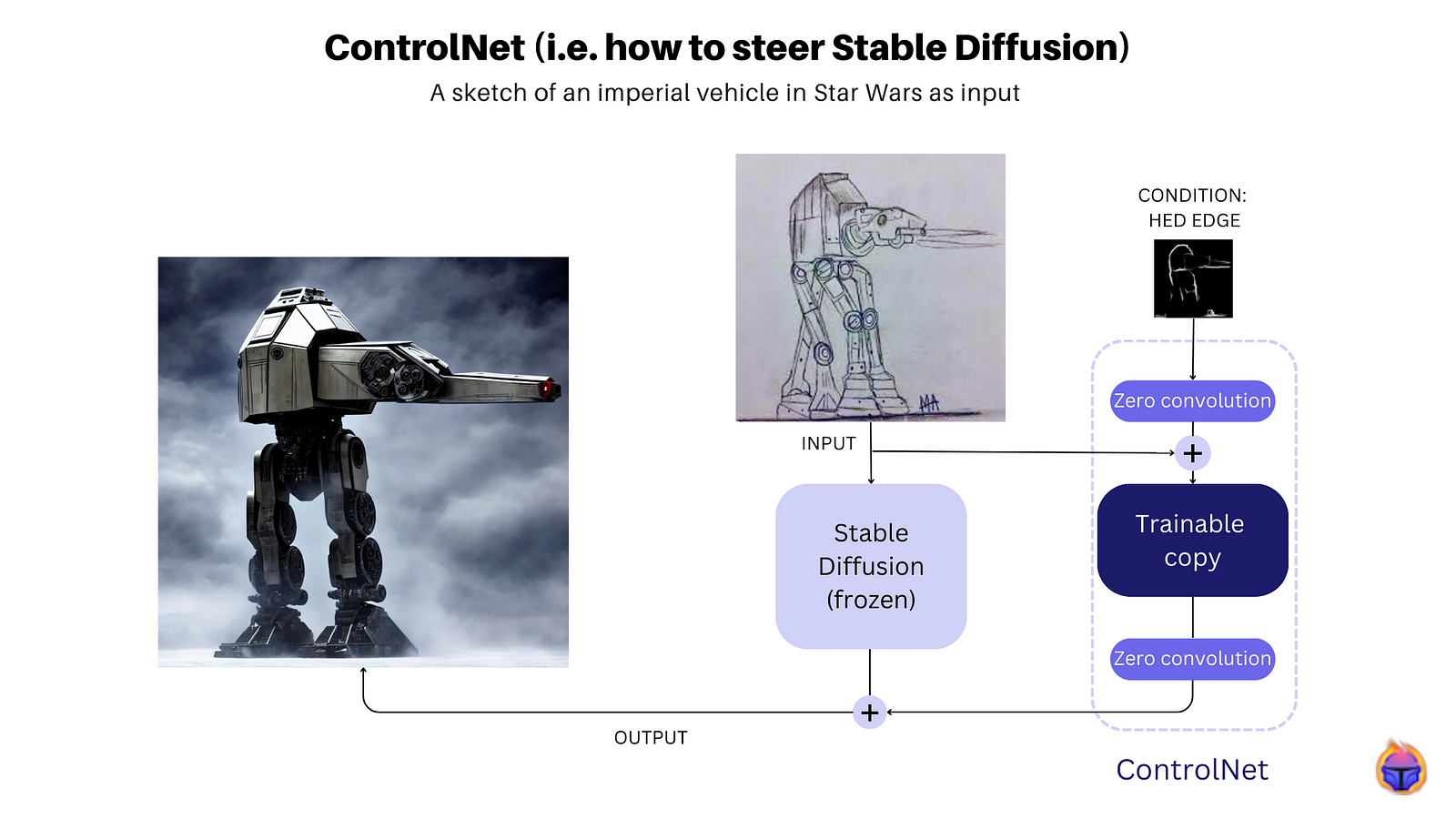
ControlNet facilitates conditional control learning for large pre-trained text-to-image diffusion models, exemplified by Stable Diffusion on Figure 2.
It accomplishes this by locking (i.e. freezing) the parameters of the large model and creating a trainable duplicate of its encoding layers, which are linked through zero convolution layers.
The novel aspect of ControlNet is its ability to treat the large pre-trained model as a robust foundational structure for acquiring various conditional controls, on Figure 2, a sketch of an imperial vehicle of Star Wars is used as input, whereas the condition is one of ControlNet’s steerable options (HED edge) without the need of a prompt. 😀 Tip: if you add a prompt you can obtain, after a few iterations, more granular results.
4. Can we use ControlNet to augment a dataset?
TL;DR: No, ControlNet is not ready to augment a dataset, some of the results are promising though! Keep reading!
Task: Based on the following production grade object detection dataset (Figure 3), see if ControlNet is capable of augmenting it. The Tenyks platform is used to showcase the current face detection dataset.
Figure 3. Face Detection dataset on the Tenyks platform
To acomplish this task we tested some open-source implementations [5], [6], [7] of ControlNet, see Figure 4.
Figure 4. ControlNet implementation available on HuggingFace [5]
As shown on Figure 5, ControlNet was able to generate images that in some cases resemble the inputs. We used two of the conditions available in ControlNet:
- Canny: simply using the edges of the input as the condition we played with the prompt to obtain a similar face but on people from different races.
- Human Pose: using the Open Pose setting of an image, we asked to generate images of women and men with different clothes. However, in this case one of the results included a non-human sample.
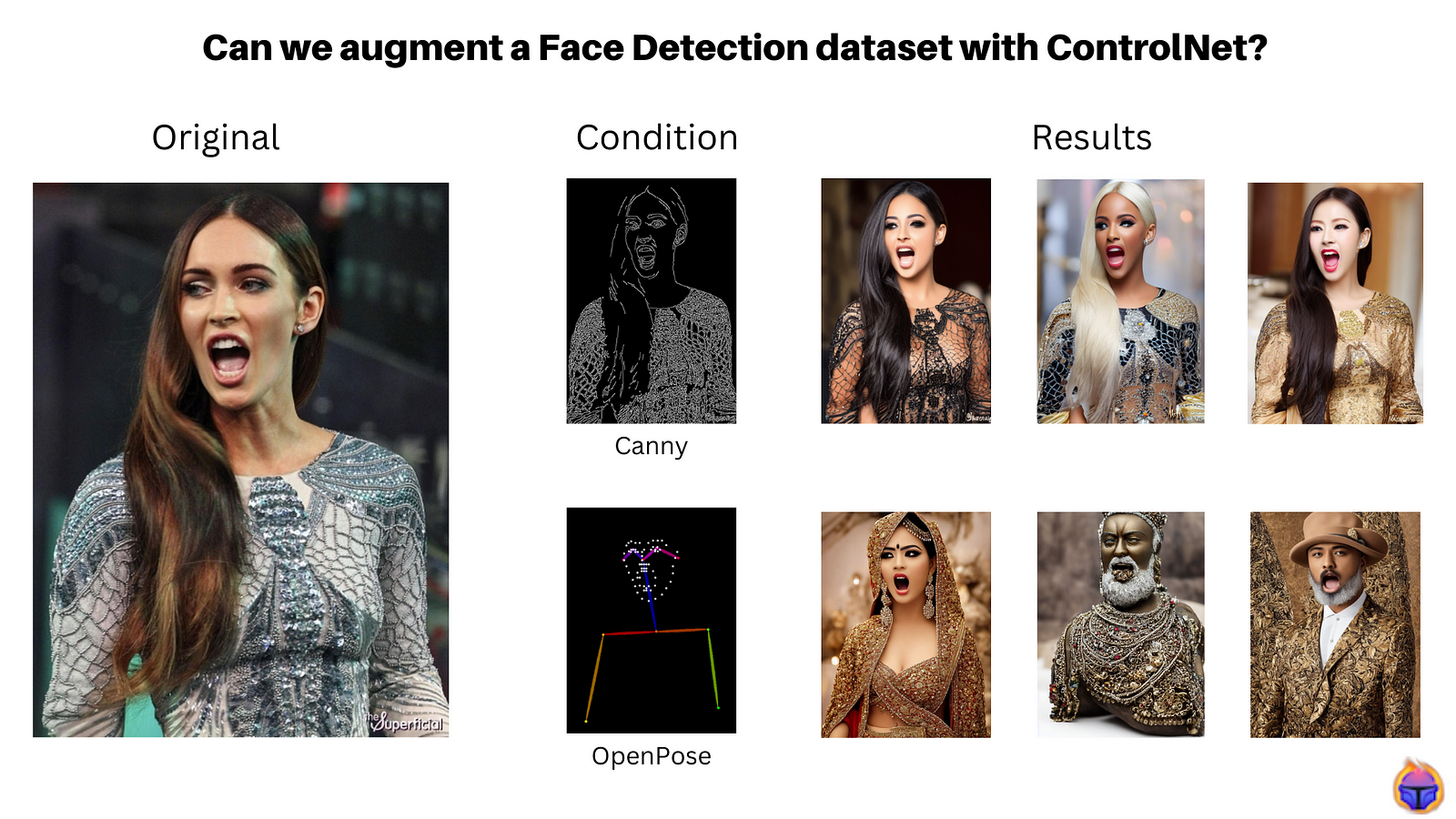
Caveat # 1: more robust implementations of ControlNet might produce better results. Caveat # 2: in fairness we didn’t do extensive prompt engineering.
5. What’s next
As we wrap up our exploration of ControlNet and its revolutionary impact on text-to-image diffusion models, it’s evident that this technology is poised to shape the future of AI-driven creativity
The journey doesn’t end here. What’s next for ControlNet? The potential applications are limitless. We can expect to see enhanced capabilities in fields such as art generation, content creation, and even medical imaging.
However, as we showed, some downstream tasks such as using Diffusion Models to generate synthetic data, require much more than finding an open source implementation.
References
[1] Stable Diffusion public release
[2] Adding Conditional Control to Text-to-Image Diffusion Models
[3] Exploring the Limitations of Stable Diffusion AI
[4] Diffusion Models: A Comprehensive Survey of Methods and Applications
[5] ControlNet v1.1
[6] ControlNet v1.1 + Anything v4.0
[7] ControlNet (pretrained weights)
Authors: Jose Gabriel Islas Montero, Dmitry Kazhdan
If you would like to know more about Tenyks, sign up for a sandbox account.
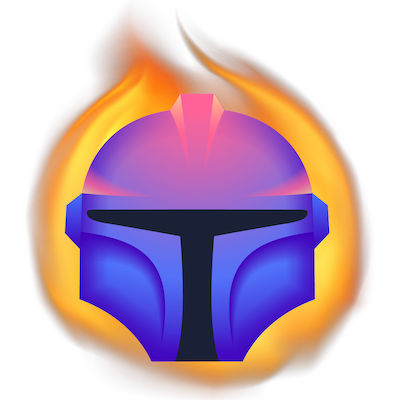